AI Development
GPT Models Can Do More Than Just Talk: How to Make Them Brew You Coffee ☕
Imagine having a GPT model that can not only generate amazing natural language, but also interact with your own data sources, APIs, or actions. How cool would that be? You could ask it to do anything you want, from booking a flight to ordering a pizza to playing a game. Well, you don't have to imagine anymore, because, in this article, I will show you how I made it happen using a simple yet powerful approach based on intent classification and a rule-based system.
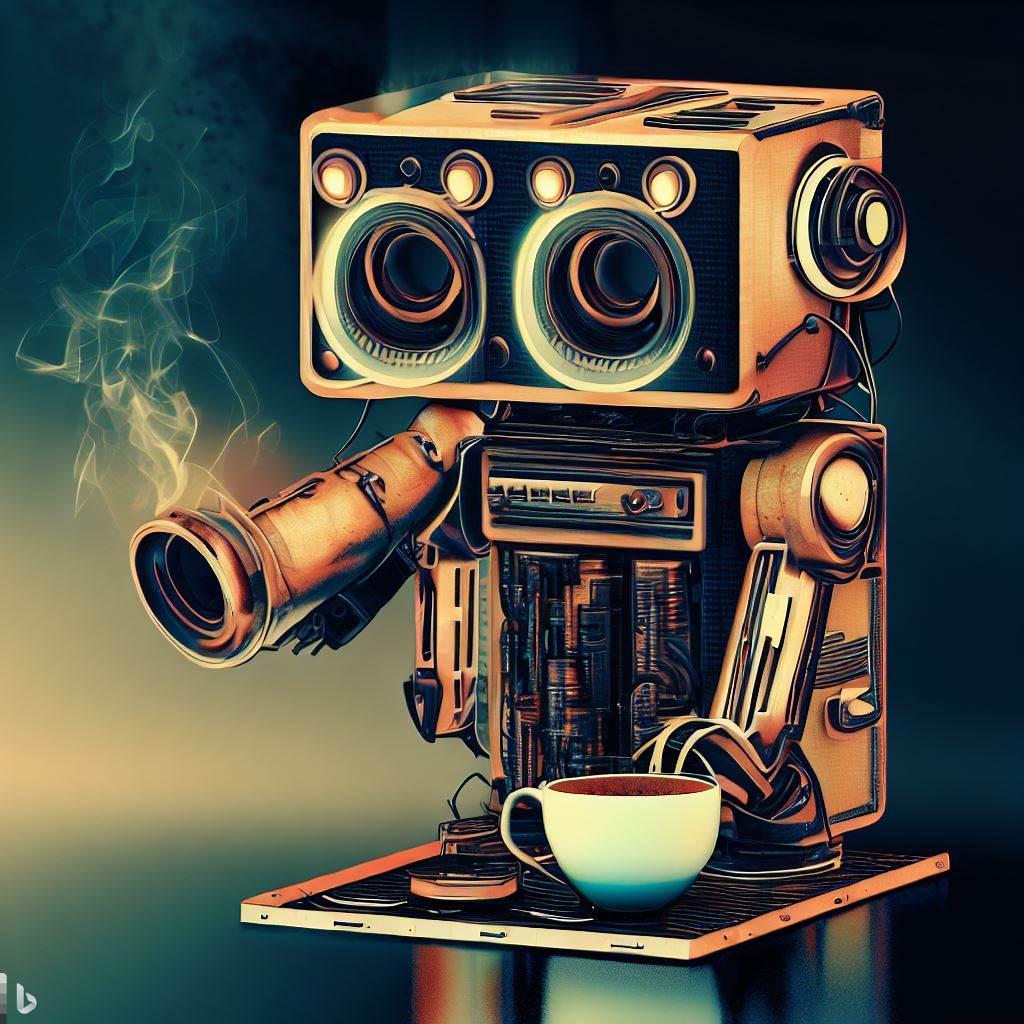
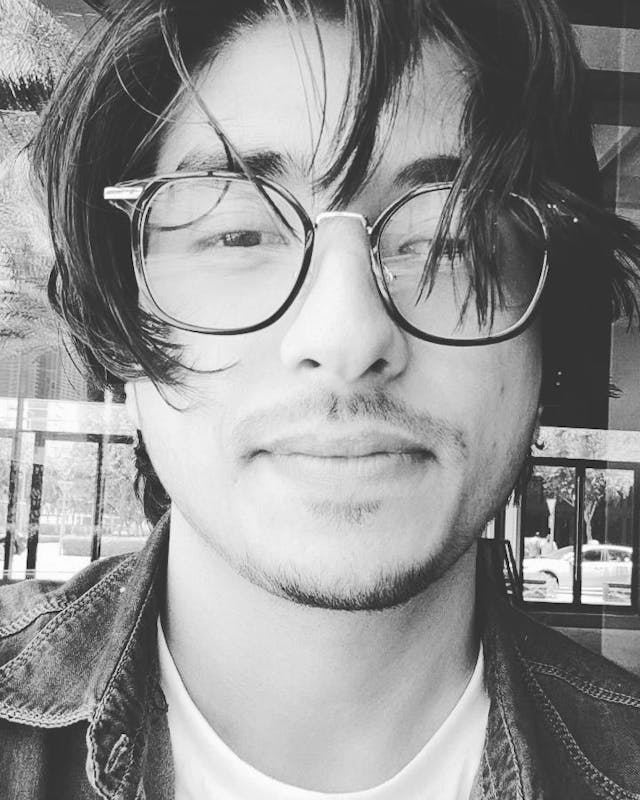
Raja Osama
I Describe Myself as a Polyglot ~ Tech Agnostic ~ Rockstar Software Engineer. I Specialise in Javascript-based tech stack to create fascinating applications.
I am also open for freelance work, and in-case you want to hire me, you can contact me at rajaosama.me@gmail.com or contact@rajaosama.me